The social world doesn’t work how we pretend it does. Too often, we are led to believe it is a structured, ordered system defined by clear rules and patterns. The economy, apparently, runs on supply-and-demand curves. Politics is a science. Even human beliefs can be charted, plotted, graphed. And using the right regression we can tame even the most baffling elements of the human condition. Within this dominant, hubristic paradigm of social science, our world is treated as one that can be understood, controlled and bent to our whims. It can’t.
Our history has been an endless but futile struggle to impose order, certainty and rationality onto a Universe defined by disorder, chance and chaos. And, in the 21st century, this tendency seems to be only increasing as calamities in the social world become more unpredictable. From 9/11 to the financial crisis, the Arab Spring to the rise of populism, and from a global pandemic to devastating wars, our modern world feels more prone to disastrous ‘shocks’ than ever before. Though we’ve got mountains of data and sophisticated models, we haven’t gotten much better at figuring out what looms around the corner. Social science has utterly failed to anticipate these bolts from the blue. In fact, most rigorous attempts to understand the social world simply ignore its chaotic quality – writing it off as ‘noise’ – so we can cram our complex reality into neater, tidier models. But when you peer closer at the underlying nature of causality, it becomes impossible to ignore the role of flukes and chance events. Shouldn’t our social models take chaos more seriously?
The problem is that social scientists don’t seem to know how to incorporate the nonlinearity of chaos. For how can disciplines such as psychology, sociology, economics and political science anticipate the world-changing effects of something as small as one consequential day of sightseeing or as ephemeral as passing clouds?
On 30 October 1926, Henry and Mabel Stimson stepped off a steam train in Kyoto, Japan and set in motion an unbroken chain of events that, two decades later, led to the deaths of 140,000 people in a city more than 300 km away.
The American couple began their short holiday in Japan’s former imperial capital by walking from the railway yard to their room at the nearby Miyako Hotel. It was autumn. The maples had turned crimson, and the ginkgo trees had burst into a golden shade of yellow. Henry chronicled a ‘beautiful day devoted to sightseeing’ in his diary.
Nineteen years later, he had become the United States Secretary of War, the chief civilian overseeing military operations in the Second World War, and would soon join a clandestine committee of soldiers and scientists tasked with deciding how to use the first atomic bomb. One Japanese city ticked several boxes: the former imperial capital. The Target Committee agreed that Kyoto must be destroyed. They drew up a tactical bombing map and decided to aim for the city’s railway yard, just around the corner from the Miyako Hotel where the Stimsons had stayed in 1926.
Stimson pleaded with the president Harry Truman not to bomb Kyoto. He sent cables in protest. The generals began referring to Kyoto as Stimson’s ‘pet city’. Eventually, Truman acquiesced, removing Kyoto from the list of targets. On 6 August 1945, Hiroshima was bombed instead.
If such random events could lead to so many deaths, how are we to predict the fates of human society?
The next atomic bomb was intended for Kokura, a city at the tip of Japan’s southern island of Kyushu. On the morning of 9 August, three days after Hiroshima was destroyed, six US B-29 bombers were launched, including the strike plane Bockscar. Around 10:45am, Bockscar prepared to release its payload. But, according to the flight log, the target ‘was obscured by heavy ground haze and smoke’. The crew decided not to risk accidentally dropping the atomic bomb in the wrong place.
Bockscar then headed for the secondary target, Nagasaki. But it, too, was obscured. Running low on fuel, the plane prepared to return to base, but a momentary break in the clouds gave the bombardier a clear view of the city. Unbeknown to anyone below, Nagasaki was bombed due to passing clouds over Kokura. To this day, the Japanese refer to ‘Kokura’s luck’ when one unknowingly escapes disaster.
Roughly 200,000 people died in the attacks on Hiroshima and Nagasaki – and not Kyoto and Kokura – largely due to one couple’s vacation two decades earlier and some passing clouds. But if such random events could lead to so many deaths and change the direction of a globally destructive war, how are we to understand or predict the fates of human society? Where, in the models of social change, are we supposed to chart the variables for travel itineraries and clouds?
In the 1970s, the British mathematician George Box quipped that ‘all models are wrong, but some are useful’. But today, many of the models we use to describe our social world are neither right nor useful. There is a better way. And it doesn’t entail a futile search for regular patterns in the maddening complexity of life. Instead, it involves learning to navigate the chaos of our social worlds.
Before the scientific revolution, humans had few ways of understanding why things happened to them. ‘Why did that storm sink our fleet?’ was a question that could be answered only with reference to gods or, later, to God. Then, in the 17th century, Isaac Newton introduced a framework where such events could be explained through natural laws. With the discovery of gravity, science turned the previously mysterious workings of the physical Universe – the changing of the tides, celestial movements, falling objects – into problems that could be investigated. Newtonian physics helped push human ideas about causality from the unknowable into the merely unknown. A world ruled by gods is fundamentally unknowable to mere mortals, but, with Newton’s equations, it became possible to imagine that our ignorance was temporary. Uncertainty could be slain with intellectual ingenuity. In 1814, for example, the French scholar Pierre-Simon Laplace published an essay that imagined the possible implications of Newton’s ideas on the limits of knowledge. Laplace used the concept of an all-knowing demon, a hypothetical entity who always knew the positions and velocities of every particle in Newton’s deterministic universe. Using this power, Laplace’s demon could process the full enormity of reality and see the future as clearly as the past.
These ideas changed how we conceived of the fundamental nature of our world. If we are the playthings of gods, then the world is fundamentally and unavoidably unruly, swayed by unseen machinations, the whims of trickster deities and their seemingly random shocks unleashed like bolts of lightning from above. But if equations are our true lords, then the world is defined by an elegant, albeit elusive, order. Unlocking the secrets of those equations would be the key to taming what only seemed unruly due to our human ignorance. And in that world of equations, reality would inevitably converge toward a series of general laws. As scientific progress advanced in the 19th and 20th centuries, Laplace’s demon became increasingly plausible. Better equations, perhaps, could lead to godlike foresight.
‘Small differences in the initial conditions produce very great ones in the final phenomena’
The search for patterns, rules and laws wasn’t limited only to the realm of physics. In biology, Darwinian principles provided a novel guide to the rise and fall of species: evolution by natural selection acted like an ordered guardrail for all life. And as the successes of the natural sciences spread, scholars who studied the dynamics of culture began to believe that the rules of biology and physics could also be used to describe the patterns of human behaviour. If there was a theoretical law for something as mysterious as gravity, perhaps there were similar rules that could be applied to the mysteries of human behaviour, too? One scholar who put such an idea in motion was the French social theorist Henri de Saint-Simon. Believing that scientific laws underpinned social behaviour, Saint-Simon proposed a more systematic, scientific approach to social organisation and governance. Social reform, he believed, would flow inexorably from scientific research. The French philosopher Auguste Comte, a contemporary of Saint-Simon and founder of the discipline of sociology, even referred to the study of human societies as ‘social physics’. It was only a matter of time, it seemed, for the French Revolution to be understood as plainly as the revolutions of the planets.
But there were wrinkles in this world of measurement and prediction, which the French mathematician Henri Poincaré anticipated in 1908: ‘it may happen that small differences in the initial conditions produce very great ones in the final phenomena. A small error in the former will produce an enormous error in the latter.’
The first of those wrinkles was discovered by the US mathematician and meteorologist Edward Norton Lorenz. Born in 1917, Lorenz was fascinated by the weather as a young boy, but he left that interest behind in the mid-1930s when he began studying mathematics at Harvard University. During these studies, the Second World War broke out and Lorenz spotted a flyer recruiting for a weather forecasting unit. He jumped at the chance to return to his childhood fascination. As the war neared its end in 1945, Lorenz began forecasting cloud cover for bombing runs over Japan. Through this work, he started to understand the severe limitations of weather prediction – forecasting was not an exact science. And so, after the war, he returned to his mathematical studies, working on predictive weather models in the hope of giving humanity a means of more accurately glimpsing the future.
One day in 1961, while modelling the weather using a small set of variables on a simple, premodern computer, Lorenz decided to save time by restarting a simulation that had been stopped halfway through. The same simulation had been run previously, and Lorenz was running it again as part of his research. He printed the variables out, then programmed the numbers back into the machine and waited for the simulation to unfold as it had before.
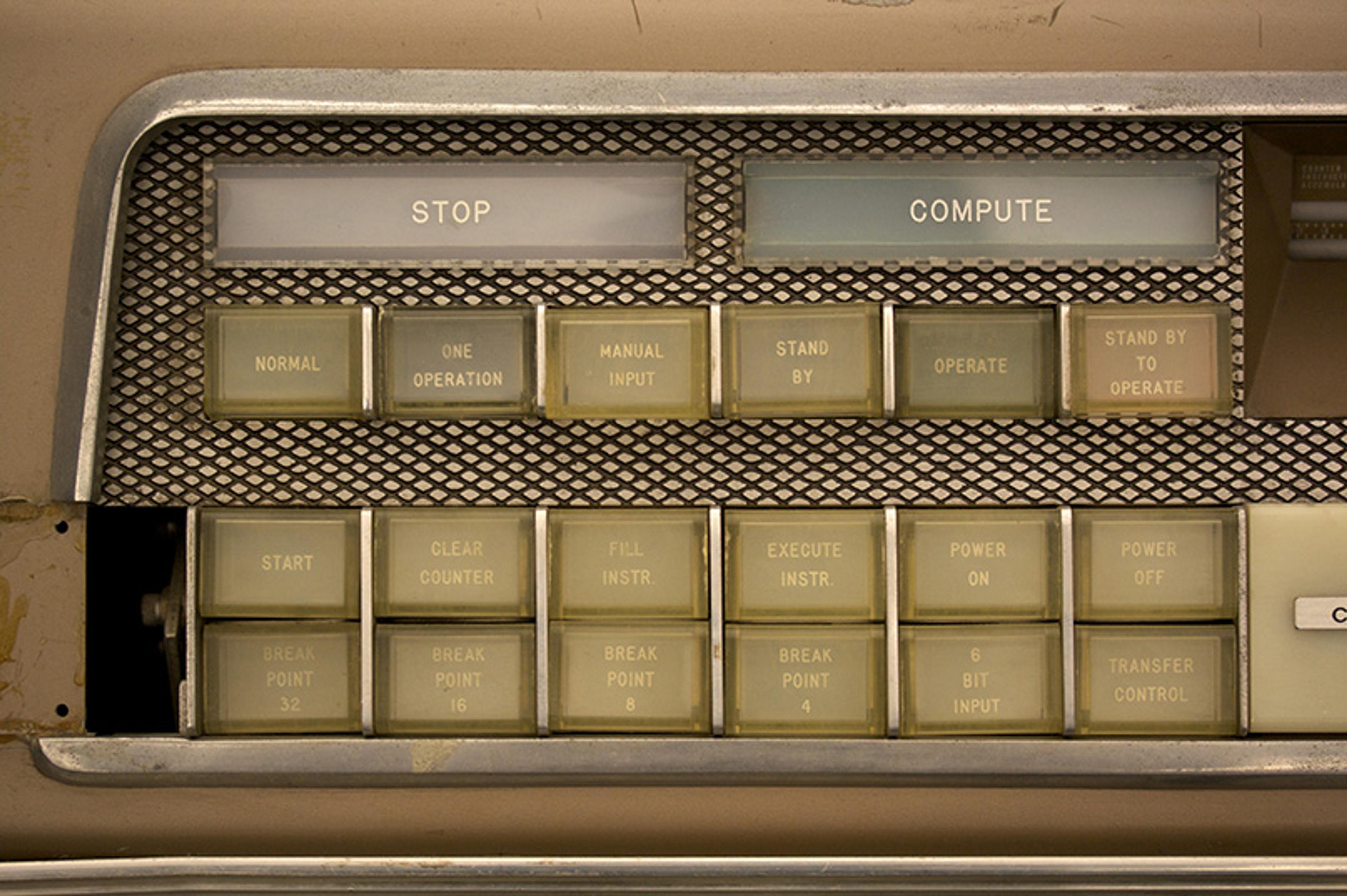
The control panel on an LGP-30 computer, similar to that used by Edward Norton Lorenz. Courtesy Wikipedia
At first, everything looked identical, but over time the weather patterns began to diverge dramatically. He assumed there must have been an error with the computer. After much chin-scratching and scowling over the data, Lorenz made a discovery that forever upended our understanding of systemic change. He realised that the computer printouts he had used to run the simulation were truncating the values after three decimal points: a value of 0.506127 would be printed as 0.506. His astonishing revelation was that the tiniest measurement differences – seemingly infinitesimal, meaningless rounding errors – could radically change how a weather system evolved over time. Tempests could emerge from the sixth decimal point. If Laplace’s demon were to exist, his measurements couldn’t just be nearly perfect; they would need to be flawless. Any error, even a trillionth of a percentage point off on any part of the system, would eventually make any predictions about the future futile. Lorenz had discovered chaos theory.

The Lorenz attractor is the iconic representation of chaos theory. Courtesy Wikipedia
The core principle of the theory is this: chaotic systems are highly sensitive to initial conditions. That means these systems are fully deterministic but also utterly unpredictable. As Poincaré had anticipated in 1908, small changes in conditions can produce enormous errors. By demonstrating this sensitivity, Lorenz proved Poincaré right.
Chaos theory, to this day, explains why our weather forecasts remain useless beyond a week or two. To predict meteorological changes accurately, we, like Laplace’s demon, would have to be perfect in our understanding of weather systems, and – no matter how advanced our supercomputers may seem – we never will be. Confidence in a predictable future, therefore, is the province of charlatans and fools; or, as the US theologian Pema Chödrön put it: ‘If you’re invested in security and certainty, you are on the wrong planet.’
Most of the genomic tweaks driving evolution are fundamentally arbitrary, even accidental
The second wrinkle in our conception of an ordered, certain world came from the discoveries of quantum mechanics that began in the early 20th century. Seemingly irreducible randomness was discovered in bewildering quantum equations, shifting the dominant scientific conception of our world from determinism to indeterminism (though some interpretations of quantum physics arguably remain compatible with a deterministic universe, such as the ‘many-worlds’ interpretation, Bohmian mechanics, also known as the ‘pilot-wave’ model, and the less prominent theory of superdeterminism). Scientific breakthroughs in quantum physics showed that the unruly nature of the Universe could not be fully explained by either gods or Newtonian physics. The world may be defined, at least in part, by equations that yield inexplicable randomness. And it is not just a partly random world, either. It is startlingly arbitrary.
Consider, for example, the seemingly ordered progression of Darwinian evolution. Alfred Russel Wallace, who discovered evolution around the same time as Charles Darwin, believed that the principles of life had a structured purpose – they were teleological. Darwin was more sceptical. But neither thinker could anticipate just how arbitrary much of evolutionary change would turn out to be.
In the 1960s, the Japanese evolutionary biologist Motoo Kimura discovered that most of the genomic tweaks driving evolution at the molecular level are neither helpful nor harmful. They are fundamentally arbitrary, even accidental. Kimura called this the ‘neutral theory of molecular evolution’. Other scientists noticed it, too, whether they were studying viruses, fruit flies, blind mole rats, or mice. Evidence began to accumulate that many evolutionary changes in species weren’t driven by structured or ordered selection pressures. They were driven by the forces of chance.
The US biologist Richard Lenski’s elegant long-term evolution experiment, which has been running since 1988, demonstrated that important adaptations that help a species (such as E coli) thrive can emerge after a chain of broadly meaningless mutations. If any one of those haphazard and seemingly ‘useless’ tweaks hadn’t occurred, the later beneficial adaptation wouldn’t have been possible. Sometimes, there’s no clear reason, no clear pattern. Sometimes, things just happen.

E coli populations from Richard Lenski’s long-term evolution experiment, 25 June 2008. Courtesy Wikipedia
Kimura’s own life was an illustration of the arbitrary forces that govern our world. In 1944, he enrolled at Kyoto University, hoping to continue his intellectual pursuits while avoiding conscription into the Japanese military. If Henry Stimson had chosen a different destination for his sightseeing vacation in 1926, Kimura and his fellow students would likely have been incinerated in a blinding flash of atomic light.
How can we make sense of social change when consequential shifts often arise from chaos? This is the untameable bane of social science, a field that tries to detect patterns and assert control over the most unruly, chaotic system that exists in the known Universe: 8 billion interacting human brains embedded in a constantly changing world. While we search for order and patterns, we spend less time focused on an obvious but consequential truth. Flukes matter.
Though some scholars in the 19th century, such as the English philosopher John Stuart Mill and his intellectual descendants, believed there were laws governing human behaviour, social science was swiftly disabused of the notion that a straightforward social physics was possible. Instead, most social scientists have aimed toward what the US sociologist Robert K Merton called ‘middle-range theory’, in which researchers hope to identify regularities and patterns in certain smaller realms that can perhaps later be stitched together to derive the broader theoretical underpinnings of human society. Though some social scientists are sceptical that such broader theoretical underpinnings exist, the most common approach to social science is to use empirical data from the past to tease out ordered patterns that point to stable relationships between causes and effects. Which variables best correlate with the onset of civil wars? Which economic indicators offer the most accurate early warning signs of recessions? What causes democracy?
Social science became dominated by one computational tool above all others: linear regressions
In the mid-20th century, researchers no longer sought the social equivalent of a physical law (like gravity), but they still looked for ways of deriving clear-cut patterns within the social world. What limited this ability was technology. Just as Lorenz was constrained by the available technology when forecasting weather in the Pacific theatre of the Second World War, so too were social scientists constrained by a lack of computing power. This changed in the 1980s and ’90s, when cheap and sophisticated computers became new tools for understanding social worlds. Suddenly, social scientists – sociologists, economists, psychologists or political scientists – could take a large number of variables and plug them into statistical software packages such as SPSS and Stata, or programming languages such as R. Complex equations would then process these data points, finding the ‘line of best fit’ using a ‘linear regression’, to help explain how groups of humans change over time. A quantitative revolution was born.
By the 2000s, area studies specialists who had previously done their research by trekking across the globe and embedding themselves in specific cultures were largely supplanted by office-bound data junkies who could manipulate numbers and offer evidence of hidden relationships that were obscured prior to the rise of sophisticated numerical analysis. In the process, social science became dominated by one computational tool above all others: linear regressions. To help explain social change, this tool uses past data to try to understand the relationships between variables. A regression produces a simplified equation that tries to fit the cluster of real-world datapoints, while ‘controlling’ for potential confounders, in the hopes of identifying which variables drive change. Using this tool, researchers can feed a model with a seemingly endless string of data as they attempt to answer difficult questions. Does oil hinder democracy? How much does poverty affect political violence? What are the social determinants of crime? With the right data and a linear regression, researchers can plausibly identify patterns with defensible, data-driven equations. This is how much of our knowledge about social systems is currently produced. There is just one glaring problem: our social world isn’t linear. It’s chaotic.
Linear regressions rely on several assumptions about human society that are obviously incorrect. In a linear equation, the size of a cause is proportionate to the size of its effect. That’s not how social change works. Consider, for example, that the assassination of one man, Archduke Franz Ferdinand, triggered the First World War, causing roughly 40 million casualties. Or think of the single vegetable vendor who lit himself on fire in central Tunisia in late 2010, sparking events that led to the Syrian civil war, resulting in hundreds of thousands of deaths and the fall of several authoritarian regimes. More recently, a bullet narrowly missed killing Donald Trump in Pennsylvania: if the tiniest gust of wind or a single bodily twitch had altered its trajectory, the 21st century would have been set on a different path. This exemplifies chaos theory in the social world, where tiny changes in initial conditions can transform countless human fates.
Another glaring problem is that most linear regressions assume that a cause-and-effect relationship is stable across time. But our social world is constantly in flux. While baking soda and vinegar will always produce a fizz, no matter where or when you mix them together, a vegetable vendor lighting himself on fire will rarely produce regional upheaval. Likewise, many archdukes have died – only one has ever triggered a world war.
Timing matters, too. Even if the exact same mutation in the exact same coronavirus had broken out in the exact same place, the economic effects and social implications of the ensuing pandemic would have been drastically different if it had struck in 1990 instead of 2020. How would millions of people have worked from home without the internet? Pandemics, like many complex social phenomena, are not uniformly governed by stable, ordered patterns. This is a principle of social reality known to economists as ‘nonstationarity’: causal dynamics can change as they are being measured. Social models often deal with this problem by ignoring it.
Most linear regressions are also ineffective at modelling two fundamental facets of our world: sequencing, the critical order in which events take place; and space, the specific physical geography in which those events occur. The overarching explanations offered by linear regression ignore the order in which things happen, and though that approach can sometimes work, at other times the order of events is crucial. Try adding flour after you bake a cake and see what happens. Similarly, linear regressions cannot easily incorporate complex features of our physical geography or capture the ways that humans navigate through space. Social models tend to conceptualise changes at the macro level, through economic output figures or democracy scores, rather than seeing diverse, adaptive individuals who are constantly interacting on specific terrain. Life looks very different for people living in Antarctica compared with people living in downtown Mumbai or the Andes or outback Australia.
We produce too many models that are often wrong and rarely useful. But there is a better way
By smoothing over near-infinite complexity, linear regressions make our nonlinear world appear to follow the comforting progression of a single ordered line. This is a conjuring trick. And to complete it successfully, scientists need to purge whatever doesn’t fit. They need to detect the ‘signal’ and delete the ‘noise’. But in chaotic systems, the noise matters. Do we really care that 99.8 per cent of the Titanic’s voyage went off without a hitch, or that Abraham Lincoln enjoyed most of the play before he was shot?
The deeply flawed assumptions of social modelling do not persist because economists and political scientists are idiots, but rather because the dominant tool for answering social questions has not been meaningfully updated for decades. It is true that some significant improvements have been made since the 1990s. We now have more careful data analysis, better accounting for systematic bias, and more sophisticated methods for inferring causality, as well as new approaches, such as experiments that use randomised control trials. However, these approaches can’t solve many of the lingering problems of tackling complexity and chaos. For example, how would you ethically run an experiment to determine which factors definitively provoke civil wars? And how do you know that an experiment in one place and time would produce a similar result a year later in a different part of the world?
These drawbacks have meant that, despite tremendous innovations in technology, linear regressions remain the outdated king of social research. As the US economist J Doyne Farmer puts it in his book Making Sense of Chaos (2024): ‘The core assumptions of mainstream economics don’t match reality, and the methods based on them don’t scale well from small problems to big problems.’ For Farmer, these methods are primarily limited by technology. They have been, he writes, ‘unable to take full advantage of the huge advances in data and technology.’
The drawbacks also mean that social research often has poor predictive power. And, as a result, social science doesn’t even really try to make predictions. In 2022, Mark Verhagen, a research fellow at the University of Oxford, examined a decade of articles in the top academic journals in a variety of disciplines. Only 12 articles out of 2,414 tried to make predictions in the American Economic Review. For the top political science journal, American Political Science Review, the figure was 4 out of 743. And in the American Journal of Sociology, not a single article made a concrete prediction. This has yielded the bizarre dynamic that many social science models can never be definitively falsified, so some deeply flawed theories linger on indefinitely as zombie ideas that refuse to die.
A core purpose of social science research is to prevent avoidable problems and improve human prosperity. Surely that requires more researchers to make predictions about the world at some point – even if chaos theory shows that those claims are likely to be inaccurate.
We produce too many models that are often wrong and rarely useful. But there is a better way. And it will come from synthesising lessons from fields that social scientists have mostly ignored.
Chaos theory emerged in the 1960s and, in the following decades, mathematical physicists such as David Ruelle and Philip Anderson recognised the significance of Lorenz’s insights for our understanding of real-world dynamical systems. As these ideas spread, misfit thinkers from an array of disciplines began to coalesce around a new way of thinking that was at odds with the mainstream conventions in their own fields. They called it ‘complexity’ or ‘complex systems’ research. For these early thinkers, Mecca was the Santa Fe Institute in New Mexico, not far from the sagebrush-dotted hills where the atomic bomb was born. But unlike Mecca, the Santa Fe Institute did not become the hub of a global movement.
Public interest in chaos and complexity surged in the 1980s and ’90s with the publication of James Gleick’s popular science book Chaos (1987), and a prominent reference from Jeff Goldblum’s character in the film Jurassic Park (1993). ‘The shorthand is the butterfly effect,’ he says, when asked to explain chaos theory. ‘A butterfly can flap its wings in Peking and in Central Park you get rain instead of sunshine.’ But aside from a few fringe thinkers who broke free of disciplinary silos, social science responded to the complexity craze mostly with a shrug. This was a profound error, which has contributed to our flawed understanding of some of the most basic questions about society. Taking chaos and complexity seriously requires a fresh approach.
One alternative to linear regressions is agent-based modelling, a kind of virtual experiment in which computers simulate the behaviour of individual people within a society. This tool allows researchers to see how individual actions, with their own motivations, come together to create larger social patterns. Agent-based modelling has been effective at solving problems that involve relatively straightforward decision-making, such as flows of car traffic or the spread of disease during a pandemic. As these models improve, with advances in computational power, they will inevitably continue to yield actionable insights for more complex social domains. Crucially, agent-based models can capture nonlinear dynamics and emergent phenomena, and reveal unexpected bottlenecks or tipping points that would otherwise go unnoticed. They might allow us to better imagine possible worlds, not just measure patterns from the past. They offer a powerful but underused tool in future-oriented social research involving complex systems.
The study of resilience in nonlinear systems would drastically improve our ability to avert avoidable catastrophes
Additionally, social scientists could incorporate chaotic dynamics by acknowledging the limits of seeking regularities and patterns. Instead, they might try to anticipate and identify systems on the brink, near a consequential tipping point – systems that could be set off by a disgruntled vegetable vendor or triggered by a murdered archduke. The study of ‘self-organised criticality’ in physics and complexity science could help social scientists make sense of this kind of fragility. Proposed by the physicists Per Bak, Chao Tang and Kurt Wiesenfeld, the concept offers a useful analogy for social systems that may disastrously collapse. When a system organises itself toward a critical state, a single fluke could cause the system to change abruptly. By analogy, modern trade networks race toward an optimised but fragile state: a single gust of wind can twist one boat sideways and cause billions of dollars in economic damage, as happened in 2021 when a ship blocked the Suez Canal.
The theory of self-organised criticality was based on the sandpile model, which could be used to evaluate how and why cascades or avalanches occur within systems. If you add grains of sand, one at a time, to a sandpile, eventually, a single grain of sand can cause an avalanche. But that collapse becomes more likely as the sandpile soars to its limit. A social sandpile model could provide a useful intellectual framework for analysing the resilience of complex social systems. Someone lighting themselves on fire, God forbid, in Norway is unlikely to spark a civil war or regime collapse. That is because the Norwegian sandpile is lower, less stretched to its limit, and therefore less prone to unexpected cascades and tipping points than the towering sandpile that led to the Arab Spring.
There are other lessons for social research to be learned from nonlinear evaluations of ecological breakdown. In biology, for instance, the theory of ‘critical slowing down’ predicts that systems near a tipping point – like a struggling coral reef that is being overrun with algae – will take longer to recover from small disturbances. This response seems to act as an early warning system for ecosystems on the brink of collapse.
Social scientists should be drawing on these innovations from complex systems and related fields of research rather than ignoring them. Better efforts to study resilience and fragility in nonlinear systems would drastically improve our ability to avert avoidable catastrophes. And yet, so much social research still chases the outdated dream of distilling the chaotic complexity of our world into a straightforward equation, a simple, ordered representation of a fundamentally disordered world.
When we try to explain our social world, we foolishly ignore the flukes. We imagine that the levers of social change and the gears of history are constrained, not chaotic. We cling to a stripped-down, storybook version of reality, hoping to discover stable patterns. When given the choice between complex uncertainty and comforting – but wrong – certainty, we too often choose comfort.
In truth, we live in an unruly world often governed by chaos. And in that world, the trajectory of our lives, our societies and our histories can forever be diverted by something as small as stepping off a steam train for a beautiful day of sightseeing, or as ephemeral as passing clouds.
Parts of this essay were adapted from Fluke: Chance, Chaos, and Why Everything We Do Matters (2024) by Brian Klaas.