Here’s the quandary when it comes to AI: have we found our way to salvation, a portal to an era of convenience and luxury heretofore unknown? Or have we met our undoing, a dystopia that will decimate society as we know it? These contradictions are at least partly due to another – somewhat latent – contradiction. We are fascinated by AI’s outputs (the what) at a superficial level but are often disenchanted if we dig a bit deeper, or otherwise try to understand AI’s process (the how). This quandary has never been so apparent as in these times of generative AI. We are enamoured by the excellent form of outputs produced by large language models (LLMs) such as ChatGPT while being worried about the biased and unrealistic narratives they churn out. Similarly, we find AI art very appealing, while being concerned by the lack of deeper meaning, not to mention concerns about plagiarising the geniuses of yesteryear.
That worries are most pronounced in the sphere of generative AI, which urges us to engage directly with the tech, is hardly a coincidence. Human-to-human conversations are layered with multiple levels and types of meanings. Even a simple question such as ‘Shall we have a coffee?’ has several implicit meanings relating to shared information about the time of the day, a latent intent to have a relaxed conversation, guesses about drink preferences, availability of nearby shops, and so on and so forth. If we see an artwork titled ‘1970s Vietnam’, we probably expect that the artist is intending to convey something about life in that country during end-war and postwar times – a lot goes unsaid while interacting with humans and human outputs. In contrast, LLMs confront us with human-like responses that lack any deeper meaning. The dissonance between human-like presentation and machine-like ethos is at the heart of the AI quandary, too.
Yet it would be wrong to think that AI’s obsession with superficial imitation is recent. The imitation paradigm has been entrenched in the core of AI right from the start of the discipline. To unpack and understand how contemporary culture came to applaud an imitation-focused technology, we must go back to the very early days of AI’s history and trace its evolution over the decades.
Alan Turing (1912-54), widely regarded as the father of AI, is credited with developing the foundational thoughts of the discipline. While AI has evolved quite dramatically over the 70 years since Turing died, one aspect of his legacy stands firmly at the heart of contemporary AI deliberations. This is the Turing test, a conceptual test that asks whether a technology can pass off its output as human.
Imagine a technology engaged in an e-chat with a human – if the technology can fool the interlocutor to believe that they are chatting with a human, it has won the Turing test. The chat-based interface that today’s LLMs use has led to the resurgence of interest in the Turing test within popular culture. Also, the Turing test is so embedded within the contemporary AI scholarly community as the ultimate test of intelligence that it may even be scandalous to say that it is only tangential to judging intelligence. Yet that’s exactly what Turing had intended in his seminal paper that first introduced the test.
Turing very evidently did not consider the imitation game a test of intelligence
It is noteworthy that Turing had called it the ‘imitation game’. Only later was it christened the ‘Turing test’ by the AI community. We don’t need to go beyond the first paragraph of Turing’s paper ‘Computing Machinery and Intelligence’ (1950) to understand the divergence between the ‘imitation game’ and judgment of whether a machine is intelligent. In the opening paragraph of this paper, Turing asks us to consider the question ‘Can machines think?’ and he admits how stumped he is.
He picks himself up after some rambling and closes the first paragraph of the paper by saying definitively: ‘I shall replace the question by another, which is closely related to it and is expressed in relatively unambiguous words.’ He then goes on to describe the imitation game, which he calls the ‘new form of the problem’. In other words, Turing is forthwith in making the point that the ‘imitation game’ is not the answer to the question ‘Can machines think?’ but is instead the form of the replaced question.
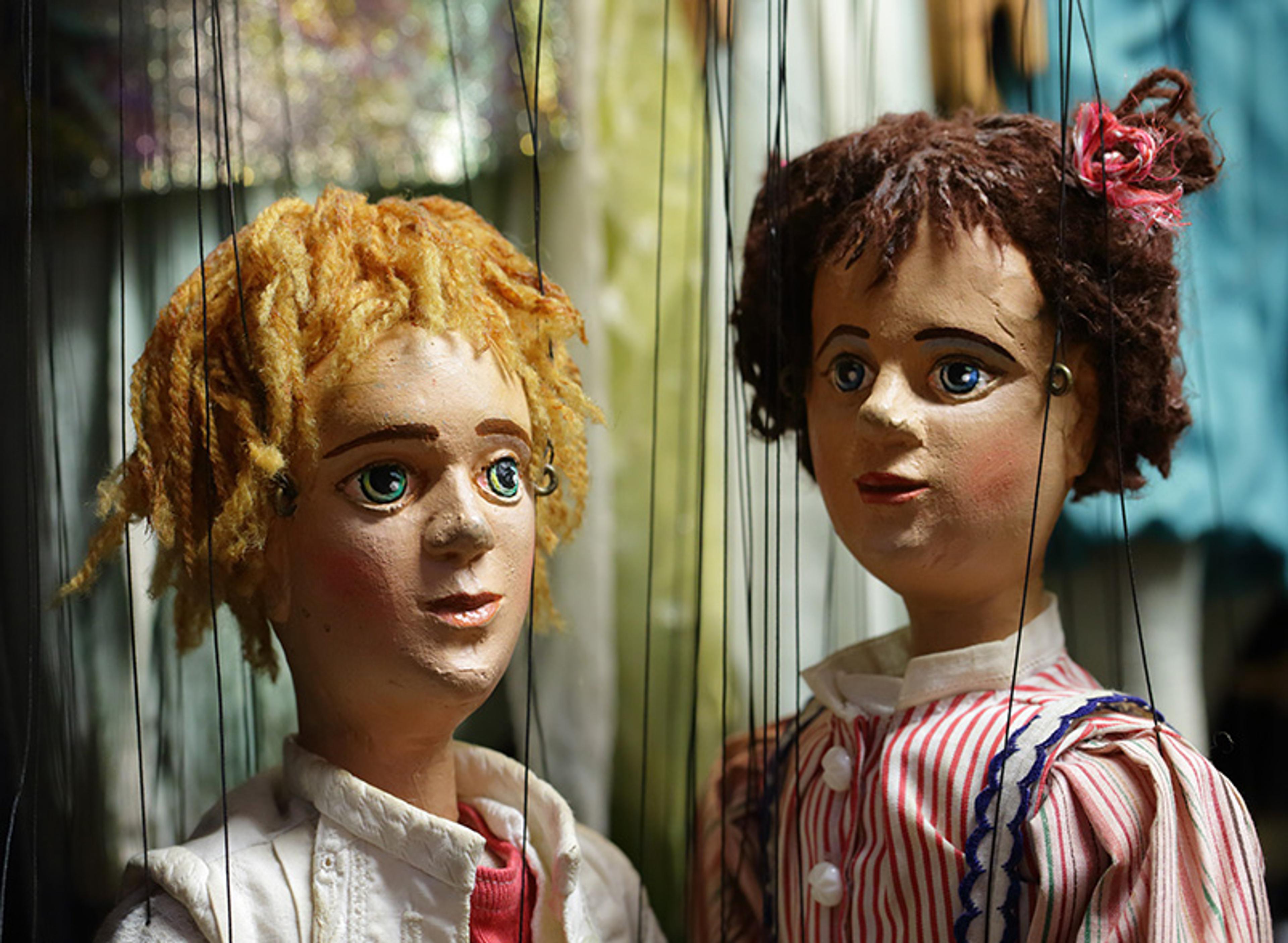
Photo by Heinz-Peter Bader/Getty
The AI community has – unfortunately, to say the least – apparently (mis)understood the imitation game as the mechanism to answer the question of whether machines are intelligent (or whether they can think or exercise intelligence). The christening of the ‘imitation game’ as the ‘Turing test’ has arguably provided an aura of authority to the test, and perhaps entrenched a reluctance in generations of AI researchers to examine it critically, given the huge following that Turing enjoys in the computing community. As recently as 2023, leaders of several nations gathered in the United Kingdom, at Bletchley Park – once Turing’s workplace – to deliberate on AI safety. In that context, the fact that Turing very evidently did not consider the imitation game a test of intelligence should offer some comfort – and courage – to engage with it critically.
Against the backdrop of Turing’s formulation of the imitation game in the early 1950s in the UK, interest was snowballing on the other side of the Atlantic around the idea of thinking machines. John McCarthy, then a young mathematics assistant professor at Dartmouth College in New Hampshire, procured funding to organise an eight-week workshop to be held during the summer of 1956. This would later be known as the ‘founding event’ of AI, and records suggest that the first substantive use of the term ‘artificial intelligence’ is in McCarthy’s funding proposal for the workshop, submitted to the Rockefeller Foundation.
For a moment, forget about ‘artificial intelligence’ as it stands now, and consider the question: what disciplines would naturally be involved in the pursuit of developing intelligent machines? It may seem natural to think that such a quest should be centred on disciplines involved with understanding and characterising intelligence as we know it – the cognitive sciences, philosophy, neuroscience, and so on. Other disciplines could act as vehicles of implementation, but the overall effort would need to be underpinned by know-how from disciplines that deal with the mind. Indeed, it was not a coincidence that Turing chose to publish his seminal paper in Mind, a journal of philosophy with substantive overlaps in the cognitive sciences. The Dartmouth workshop was notably funded by the Biological and Medical Research division of the Rockefeller Foundation, reflecting that the above speculations may not be off the mark. Yet McCarthy’s workshop was radically different in structure.
Mathematical researchers no longer had to feel alone when talking about thinking machines as computing
The Dartmouth workshop was dominated by mathematicians and engineers, including a substantive participation from technology companies such as IBM; there was little presence of scholars from other disciplines. A biographical history comprising notes by Ray Solomonoff, a workshop participant, and compiled by his wife Grace Solomonoff, provides ample evidence that the ‘artificial intelligence’ project was actively shunted along the engineering direction and away from the neuro-cognitive-philosophical direction. In particular, Solomonoff’s notes record one of the core organisers, Marvin Minsky, who would later become a key figure in AI, opining thus in a letter in the run-up to the workshop:
[B]y the time the project starts, the whole bunch of us will, I bet, have an unprecedented agreement on philosophical and language matters so that there will be little time wasted on such trivialities.
It could be that other participants shared Minsky’s view of philosophical and language matters as time-wasting trivialities, but not voiced them as explicitly (or as bluntly).
In a description of discussions leading up to the workshop, the historian of science Ronald Kline shows how the event, initially conceptualised with significant space for pursuits like brain modelling, gradually gravitated towards a mathematical modelling project. The main scientific outcome from the project, as noted in both Solomonoff’s and Kline’s accounts, was to establish mathematical symbol manipulation – what would later be known as symbolic AI – as the pathway through which AI would progress. This is evident when one observes that, two years later, in a 1958 conference titled ‘Mechanisation of Thought Processes’ (a name that would lead any reader to think of it as a neuro-cognitive-philosophical symposium), many participants of the Dartmouth workshop would present papers on mathematical modelling.
The titles of the workshop papers range from ‘heuristic programming’ to ‘conditional probability computer’. With the benefit of hindsight, one may judge that the Dartmouth workshop bolstered the development of thinking machines as an endeavour located in engineering and mathematical sciences, rather than one to be led by ideas from disciplines that seek to understand intelligence as we know it. With the weight of the Dartmouth scholars behind them, mathematical researchers no longer had to feel alone, apologetic or defensive when talking about thinking machines as computing – the sidelining of social sciences in the development of synthetic intelligence had been mainstreamed.
Yet the question remains: how could a group of intelligent people be convinced that the pursuit of ‘artificial intelligence’ shouldn’t waste time on philosophy, language and, of course, other aspects such as cognition and neuroscience? We can only speculate, again with the benefit of hindsight, that this was somehow fallout from a parochial interpretation of the Turing test, an interpretation enabled by developments in Western thought over four to five centuries. If you are one who believes that ‘thinking’ or ‘intelligence’ is possible only within an embodied and living organism, it would be absurd to ask ‘Can machines think?’ as Turing did, in his seminal paper.
Thus, even envisioning synthetic intelligence as a thing requires one to believe that intelligence or thinking can exist outside an embodied, living organism. René Descartes, the 17th-century philosopher who is embedded in contemporary popular culture through the pervasively recognised quote ‘I think, therefore, I am’, posited that the seat of thought in the human body is the mind, and that the body cannot think. This idea, called Cartesian mind-body dualism, establishes a hierarchy between the mind (the thinking part) and the body (the non-thinking part) – marking a step towards localising intelligence within the living organism.
The high-level project of synthetic intelligence has no natural metric of success
Not long after Descartes’s passing, on the other side of the English Channel, another tall philosopher, Thomas Hobbes, would write in his magnum opus Leviathan (1651) that ‘reason … is nothing but reckoning’. Reckoning is to be interpreted as involving mathematical operations such as addition and subtraction. Descartes and Hobbes had their substantive disagreements – yet their ideas synergise well; one localises the thinking in the mind, and the other reductively characterises thinking as computation. The power of the synergy is evident in the thoughts of Gottfried Leibniz, a philosopher who was probably acquainted with Descartes’s dualism and Hobbes’s materialism as a young adult, and who took the reductionist view of human thought further still. ‘When there are disputes among persons,’ he wrote in 1685, ‘we can simply say, “Let us calculate,” and without further ado, see who is right.’ For Leibniz, everything can be reduced to computation. It is against this backdrop of Western thought that Turing would – three centuries later – ask the question ‘Can machines think?’ It is notable that such ideas are not without detractors – embodied cognition has seen a revival lately, but still remains in the fringes.
While centuries of such philosophical substrate provide a fertile ground for imagining synthetic intelligence as computation, a mathematical or engineering project towards developing synthetic intelligence cannot take off without ways of quantifying success. Most scientific and engineering quests come with natural measures of success. The measure of success in developing aircraft is to see how well it can fly – how long, how high, how stably – all amenable to quantitative measurements. However, the high-level project of synthetic intelligence has no natural metric of success. This is where the ‘imitation game’ provided a much-needed take-off point; it asserted that success in developing artificial intelligence can simply be measured by whether it can generate intelligent-looking outputs that could pass off as a human’s.
Analogous to how Descartes’s ideas suggested that we need not bother about the body while exploring thinking, and in a similar reductive spirit, the structure of the ‘imitation game’ suggested that artificial intelligence need not bother about the process (the how) and could just focus on the output (the what). This dictum has arguably shaped artificial intelligence ever since; if a technology can imitate humans well, it is ‘intelligent’.
Having established that imitation is sufficient for intelligence, the AI community has a natural next target. The Turing test says that the human should be tricked by the technology into believing she is interacting with another human to prove intelligence, but that criterion is abstract, qualitative and subjective. Some humans may be more adept than others in picking up subtle signs of a machine, much like some contemporary factcheckers have the knack of spotting that little evidence of inauthenticity in deep fakes. The AI community must find reliable pathways towards developing technological imitations that could be generally regarded as intelligent by humans – in simple terms, there needs to be a generalisable structure adequate to reliably feigning intelligence. This is evident in McCarthy’s own words in 1983, when he characterises AI as the ‘science and engineering of making computers solve problems and behave in ways generally considered to be intelligent’ – of the two things, the former is not novel, the latter is. We will look at two dominant pathways across the 1960s to the ’80s that powered the quest for AI’s advancement through designing imitation technology.
In the 1960s, Joseph Weizenbaum developed a simple chatbot within the domain of Rogerian psychotherapy, where the idea is to encourage the patient to think through their condition themselves. The chatbot, named ELIZA, would use simple transformation rules, often just to put the onus back on the human; while very unlike LLMs in its internals, the emergence of LLMs has led to narratives comparing and contrasting the two.
‘When part of a mechanism is concealed from observation, the behaviour of the machine seems remarkable’
An example transformation, from Weizenbaum’s own paper about the system, involves responding to ‘I am (X)’ by simply making the chatbot ask ‘How long have you been (X)?’ Notwithstanding the simple internal processing, ELIZA’s users, to Weizenbaum’s amusement, often mistook it to be human.
‘Some subjects have been very hard to convince that Eliza (with its present script) is not human,’ wrote Weizenbaum (italics original), in an article published in 1966 in Communications of the ACM, among the foremost journals in computing.
This resonates with a general observation, which may sound prophetic in hindsight, made by Ross Ashby at the Dartmouth conference: ‘When part of a mechanism is concealed from observation, the behaviour of the machine seems remarkable.’
Today, the ELIZA effect is used to refer to the category of mistake in which symbol manipulation is mistaken for cognitive capability. Several years later, the cognitive scientist Douglas Hofstadter would call the ELIZA effect ‘ineradicable’, suggesting that a gullibility intrinsic to humans could be adequate for AI’s goals. The ELIZA effect – or the adequacy of opaque symbol manipulation to sound intelligent to human users – would turbocharge AI for the next two or three decades to come.
The wave of symbolic AI led to the development of several AI systems – often called ‘expert systems’ – that were powered by symbol manipulation rulesets of varying sizes and complexity. One of the major successes was a system developed at Stanford University in the 1970s called MYCIN, powered by around 600 rules and designed to recommend antibiotics (many of which end in -mycin, hence the name). One of AI’s main 20th-century successes, the victory by IBM’s Deep Blue chess-playing computer over the reigning (human) world champion in 1997, was also based on the success of rule-based symbolic AI.
While opaque symbolic AI has been widespread, there has been a second high-level mechanism that was found to be useful to create a pretence of intelligence. As a step towards understanding that, consider a simple thermometer or a pressure gauge – these are intended to measure temperature and pressure. They obviously have nothing to do with ‘intelligence’ per se.
But let’s now connect a simple decision mechanism to the thermometer: if the temperature goes above a preset threshold, it switches on the air conditioner (and vice versa). These little regulating mechanisms, often called thermostats, are pervasive in today’s electronic devices, whether it be ovens, water heaters, air conditioners, and even used within computers to prevent overheating. Cybernetics, the field involving feedback-based devices such as thermostats and their more complex cousins, was widely regarded as a pathway towards machine intelligence. Grace Solomonoff records ‘cybernetics’ as a potential name considered by McCarthy for the Dartmouth workshop (in lieu of the eventual ‘artificial intelligence’); the other being ‘automata theory’. The key point here is that the sense-then-respond mechanism of self-regulation employed within the likes of a thermostat could seem like some form of intelligence. We can only speculate on the reasons why we might think so; perhaps it’s because we consider sensing to be very intrinsically connected with being human (the loss of sensory capacity – even simply the loss of taste, which most of us experienced during COVID-19 – can be very impoverishing), or because the body maintains homoeostasis, among the most complex versions of life-sustaining self-regulation.
McCarthy could be seen talking about a thermostat’s beliefs, and even extending the logic to automated tellers
Yet we’re not likely to confuse simple thermostats for thinking machines, are we? Well, as long as we don’t think as McCarthy did. More than two decades after the Dartmouth workshop, its pioneering organiser would go on to write in the article ‘Ascribing Mental Qualities to Machines’ (1979) that thermostats had beliefs.
He writes: ‘When the thermostat believes the room is too cold or too hot, it sends a message saying so to the furnace.’ At parts in the article, McCarthy seems to recognise that there would naturally be critics who would ‘regard attribution of beliefs to machines as mere intellectual sloppiness’, but he goes on to say that ‘we maintain … that the ascription is legitimate.’
McCarthy admits that thermostats don’t have deeper forms of belief such as introspective beliefs, viz ‘it doesn’t believe that it believes the room is too hot’– a great concession indeed! In academia, some provocative pieces tend to be written just out of enthusiasm and convenience, especially when caught off guard. A reader who has seen bouts of unwarranted enthusiasm leading to articles may find it reasonable to urge that McCarthy’s piece shouldn’t be over-read – perhaps, it was just a one-off argumentation.
Yet, historical records tell us that’s not the case; four years later, McCarthy would write the piece ‘The Little Thoughts of Thinking Machines’ (1983). In that paper, he could be seen talking about a thermostat’s beliefs, and even extending the logic to automated tellers, which was probably starting to become an amusing piece of automation around that time. He writes: ‘The automatic teller is another example. It has beliefs like, “There’s enough money in the account,” and “I don’t give out that much money”.’
Today, the sense-then-respond mechanism powers robots extensively, with humanoid robots dominating the depiction of artificial intelligence in popular imagery, as can be seen with a quick Google image search. The usage of the adjective smart to refer to AI systems could be seen as correlated with an abundance of sense-then-response mechanisms: smart wearables involve sensors deployed at a person-level, smart homes are homes with several interconnected sensors all over the home, and smart cities are cities with abundant sensor-based surveillance. The new wave of sensor-driven AI, often referred to as ‘internet of things’, is powered by sensors.
Opaque symbolic AI and sensor-driven cybernetics are useful pathways to design systems that behave in ways generally considered to be intelligent, but we still ought to expend the effort to design these systems. Does the design requirement pose hurdles? This question leads us to the next epoch in AI scholarship.
A rapidly expanding remit of AI started experiencing some strong headwinds in certain tasks, around the 1980s. This is best captured by Hans Moravec’s book Mind Children (1988) in what has come to be known as Moravec’s paradox:
[I]t is comparatively easy to make computers exhibit adult-level performance on intelligence tests or playing checkers, and difficult or impossible to give them the skills of a one-year-old when it comes to perception and mobility.
The AI that had started to excel at checkers and chess through symbolic methods wasn’t able to make progress in distinguishing handwritten characters or identifying human faces. Such tasks are what may fall within a category of innately human (or, well, animal) activities – something we do instantly and instinctively but can’t explain how. Most of us can instantly recognise emotions from people’s faces with a high degree of accuracy – but won’t be enthusiastic about taking up a project to build a set of rules to recognise emotions from people’s images. This relates to what is now known as Polanyi’s paradox: ‘We can know more than we can tell’ – we rely on tacit knowledge that often can’t be verbally expressed, let alone be encoded as a program. The AI bandwagon has hit a brick wall.
A rather blunt (and intentionally provocative) analogy might serve well here, to understand how AI scholarship wiggled out of this conundrum. In school, each of us had to attempt and pass exams to illustrate our understanding of the topic and achievement of learning outcomes. Yet some students are too lazy to undertake the hard work; they simply copy from the answer sheets of their neighbours in the exam hall.
We call this cheating or, in milder and more sophisticated terms, academic malpractice. To complete the analogy, our protagonist is the Turing test, and the AI scholarship is not lazy, but has run out of ways to expand to address tasks that we do based on tacit knowledge. It is simply incompetent. If the reader would forgive the insinuating tone, I observe here that AI took the same pathway as the lazy student: copying from others – in this case, from us humans.
Crude models are lazy learners; deep learning models are eager learners
To really see this copying paradigm, consider a simple task, that of identifying faces in images. For humans, it is an easy perception task. We see an image and instantly recognise the face within it, if any – we almost can’t not do this task each time we see a picture (try it). Blinking an eye would take more time.
If you entrust an AI engineer to do this today, they wouldn’t think twice about adopting a data-driven or machine-learning methodology to undertake it. It starts by gathering a number of images and having human annotators label them – does each contain a face or not? This leads to a collection of two stacks of images; one with faces, another without. The labelled images would be used to train the machines, and that is how those machines learn to make the match.
This labelled dataset of images is called the training data. The more sophisticated the machine learning model, the more images and rules and operations it would use to decide whether another picture in front of it contains a face or not. But the fundamental paradigm is that of copying from labelled data mediated by a statistical model, where the statistical model could be as simple as a similarity, or it could be a very complex and carefully curated set of ‘parameters’ (as in deep learning models, which are more fashionable in current times).
Crude models are lazy learners because they don’t consult the training data until called upon to make a decision, whereas deep learning models are eager learners because they distil the training data into statistical models upfront, so that decisions can be made fast.
While there is enormous complexity and variety in the types of tasks and decision-making models, the fundamental principle remains the same: similar data objects are useful for similar purposes. If machine learning had a church, the facade could sport the dictum (in Latin, as they do for churches): Similia objectum, similia proposita. If you’re curious what this means, please consult a data-driven AI that’s specialised for language translation.
The availability of LLMs since the release of ChatGPT in late 2022 heralded a global wave of AI euphoria that continues to this day. It was often perceived in popular culture as a watershed moment, which indeed it could be, on a social level, since AI never before pervaded public imagination as it does now. Yet, at a technical level, LLMs have machine learning at their core and are technologically generating a newer form of imitation – an imitation of data; this contrasts with the conventional paradigm involving imitation of human decisions on data.
Through LLMs, imitation has taken a newer and more generalised form – it is presented as an all-knowing person, always available to be consulted on anything under the sun. Yet, it follows the same familiar copying pathway that is entrenched at the core of machine learning. As the prominent AI researcher Emily Bender and fellow AI ethicists would argue, these are ‘stochastic parrots’; while parrots that simply repeat what they hear are impressive in their own right, randomised – or stochastic – query-dependent and selective reproductions of training data have been discovered as a paradigm to create a pretence of agency and, thus, of intelligence. The reader may remember that the heuristics of opaque symbol manipulation and sensor-driven cybernetics had their heydays in the 1960s and ’70s – now, it is the turn of randomised data copying.
It is evident that biases and hallucinations are features, not bugs
The much-celebrated value of LLMs is in generating impeccable outputs: pleasing and well-written text. One may wonder how LLMs generate well-formed text when so much of the text on the web is not of such good quality, and may even imagine that to be an intrinsic merit of the technology. This is where it becomes interesting to understand how LLMs piggyback on various forms of human input. It has been noted that the most popular commercial LLM, ChatGPT, employed thousands of low-paid annotators in Kenya to grade the quality of human text and, in particular, to exclude ones regarded as toxic. Thus, the observed higher quality of LLM text is also an artefact and output of the imitation paradigm rooted in the core of AI.
Once you understand this, it’s easier to understand why LLMs could produce substantially biased outputs, including those on gender and racial lines, as noted in recent research. The randomised data-copying paradigm involves mixing and matching patterns from different parts of training data – these create narratives that don’t gel well, and consequently yield embarrassingly absurd and illogical text, often referred to as ‘hallucinations’. Understanding LLMs as imitation on steroids, it is evident that biases and hallucinations are features, not bugs. Today, the success of the LLM has spilled over to other kinds of data to herald the advent of generative AI that encompasses image and video generation, all of which are infested with issues of bias and hallucinations, as may be expected.
Let’s take an adversarial position to the narrative so far. Artificial intelligence, as it stands today, may be designed to produce imitations to feign intelligence. Yet if it does the job, why obsess ourselves with nitpicking?
This is where things get a bit complicated, but very interesting. Consider a radiologist trained in diagnosing diseases from X-rays. Their decision is abundantly informed by their knowledge of human biology. We can get many such expert radiologists to label X-rays with the diagnosis. Once there are enough X-ray diagnosis pairs, these can be channelled into a data-driven AI, which can then be used to diagnose new X-rays. All good. The scene is set for some radiologists to receive redundancy letters.
Years pass.
As luck would have it, the world is hit by COVID-27, a respiratory pandemic of epic proportions, like its predecessor. The AI knows nothing of COVID-27, and thus, can’t diagnose the disease. Having pushed many radiologists into other sectors, we no longer have enough experts to diagnose. The AI knows nothing about human biology, and its ‘knowledge’ can’t be repurposed for COVID-27 – but there is an abundance of X-rays labelled for COVID-27, encompassing all its variants, to retrain the statistical model.
The same AI that pushed radiologists out of their jobs is now in need of those very same folks to ‘teach’ it to imitate decisions about COVID-27. Even if no COVID-27 comes, viruses mutate, diseases change, the world never remains static. The AI model is always at risk of becoming stale. Thus, a continuous supply of human-labelled data is the lifeblood of data-driven AI, if it is to remain relevant to changing times. This intricate dependency on data is a latent aspect of AI, which we often underestimate at risk of our own eventual peril.
The statistical models of AI codify our biases and reproduce them with a veneer of computational objectivity
Replace radiology with policing, marking university assessments, hiring, and even making decisions on environmental factors such as weather prediction, or genAI applications such as video generation and automated essay writing, and the high-level logic remains the same. The paradigm of AI – interestingly characterised by the popular AI critic Cathy O’Neil in Weapons of Math Destruction (2016) as ‘project[ing] the past into the future’ – simply doesn’t work for fields that change or evolve. At this juncture, we may do well to remember Heraclitus, the Greek philosopher who lived 25 centuries back – he would quip that ‘change is the only constant’.
As the historian Yuval Noah Harari would say, belief that AI knows all, that it is truly intelligent and has come to save us, promotes the ideology of ‘dataism’, which is the idea of assigning supreme value to information flows. Further, given that human labelling – especially in social decision-making such as policing and hiring – is biased and ridden with stereotypes of myriad shades (sexism, racism, ageism and others), the statistical models of AI codify these biases and reproduce them with a veneer of computational objectivity. Elucidating the nature of finer relationships between the paradigm of imitation and AI’s bias problem is a story for another day.
If imitations are so problematic, what are they good for? Towards understanding this, we may take a leaf out of Karl Marx’s scholarship on the critique of the political economy of capital, capital understood as the underpinning ethos of the exploitative economic system that we understand as capitalism. Marx says that capital is concerned with the utilities of objects only insofar as they have the general form of a commodity and can be traded in markets to further monetary motives. In simple terms, towards advancing profits, efforts to improve the presentation – through myriad ways such as packaging, advertising and others – would be much more important than efforts to improve the functionality (or use-value) of the commodity.
The subordination of content to presentation is thus, unfortunately, the trend in a capitalist world. Extending Marx’s argument to AI, the imitation paradigm embedded within AI is adequate for capital. Grounded on this understanding, the interpretation of the imitation game – err, the Turing test – as a holy grail of AI is hand-in-glove with the economic system of capitalism. From this vantage point, it is not difficult to see why AI has synergised well with the markets, and why AI has evolved as a discipline dominated by big market players such as Silicon Valley’s tech giants. This market affinity of AI was illustrated in a paper that showed how AI research has been increasingly corporatised, especially when the imitation paradigm took off with the emergence of deep learning.
The wave of generative AI has set off immense public discourse on the emergence of real artificial general intelligence. However, understanding AI as an imitation helps us see through this euphoria. To use an overly simplistic but instructive analogy, kids may see agency in imitation apps like My Talking Tom – yet, it is obvious that a Talking Tom will not become a real talking cat, no matter how hard the kid tries. The market may give us sophisticated and intelligent-looking imitations, but these improvements are structurally incapable of taking the qualitative leap from imitation to real intelligence. As Hubert Dreyfus wrote in What Computers Can’t Do (1972), ‘the first man to climb a tree could claim tangible progress toward reaching the moon’ – yet, actually reaching the Moon requires qualitatively different methods than tree-climbing. If we are to solve real problems and make durable technological progress, we may need much more than an obsession with imitations.