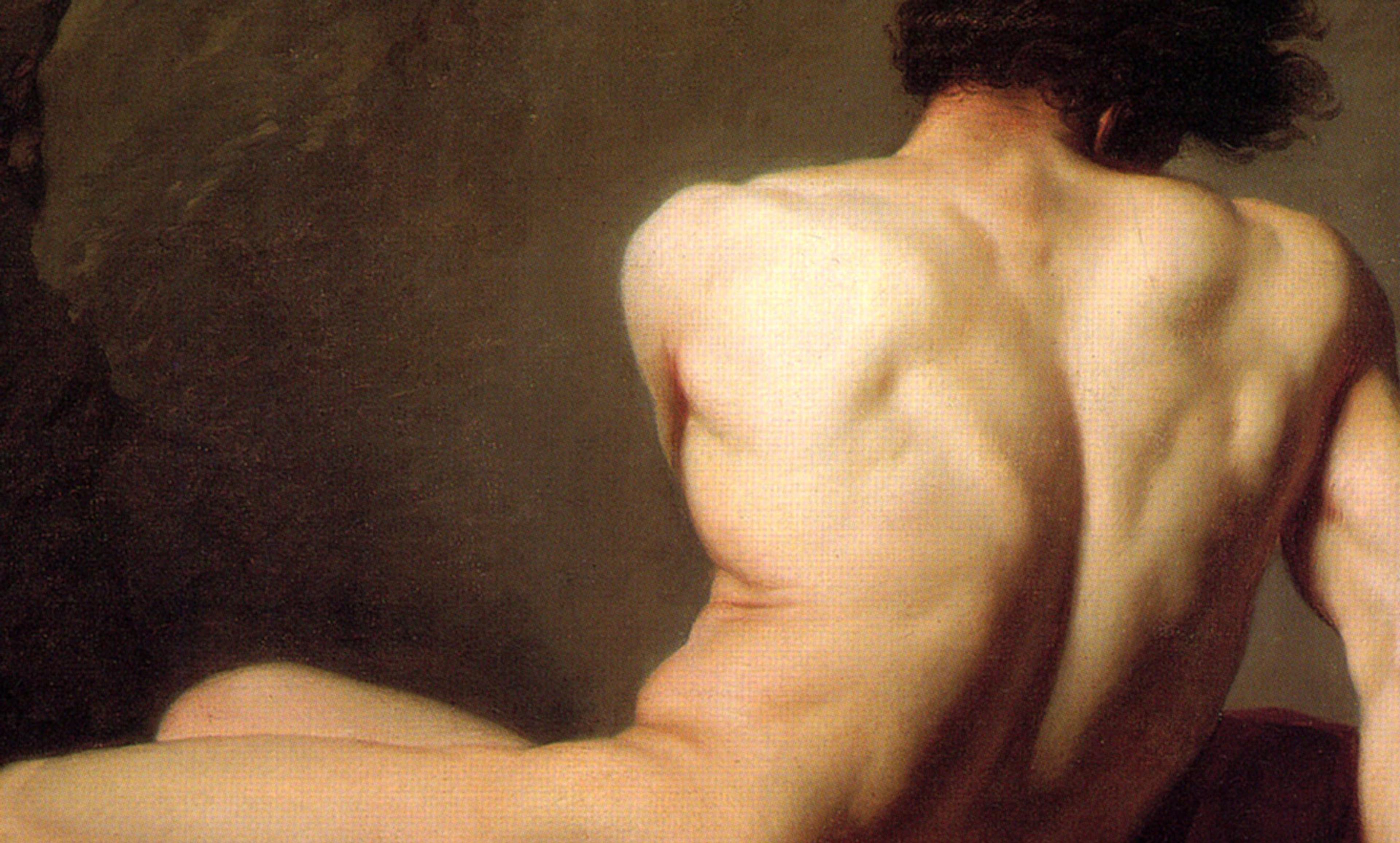
Detail from Patroclus by Jacques Louis David, 1780. Courtesy Wikipedia
It’s tempting to think of the mind as a layer that sits on top of more primitive cognitive structures. We experience ourselves as conscious beings, after all, in a way that feels different to the rhythm of our heartbeat or the rumblings of our stomach. If the operations of the brain can be separated out and stratified, then perhaps we can construct something akin to just the top layer, and achieve human-like artificial intelligence (AI) while bypassing the messy flesh that characterises organic life.
I understand the appeal of this view, because I co-founded SwiftKey, a predictive-language software company that was bought by Microsoft. Our goal is to emulate the remarkable processes by which human beings can understand and manipulate language. We’ve made some decent progress: I was pretty proud of the elegant new communication system we built for the physicist Stephen Hawking between 2012 and 2014. But despite encouraging results, most of the time I’m reminded that we’re nowhere near achieving human-like AI. Why? Because the layered model of cognition is wrong. Most AI researchers are currently missing a central piece of the puzzle: embodiment.
Things took a wrong turn at the beginning of modern AI, back in the 1950s. Computer scientists decided to try to imitate conscious reasoning by building logical systems based on symbols. The method involves associating real-world entities with digital codes to create virtual models of the environment, which could then be projected back onto the world itself. For instance, using symbolic logic, you could instruct a machine to ‘learn’ that a cat is an animal by encoding a specific piece of knowledge using a mathematical formula such as ‘cat > is > animal’. Such formulae can be rolled up into more complex statements that allow the system to manipulate and test propositions – such as whether your average cat is as big as a horse, or likely to chase a mouse.
This method found some early success in simple contrived environments: in ‘SHRDLU’, a virtual world created by the computer scientist Terry Winograd at MIT between 1968-1970, users could talk to the computer in order to move around simple block shapes such as cones and balls. But symbolic logic proved hopelessly inadequate when faced with real-world problems, where fine-tuned symbols broke down in the face of ambiguous definitions and myriad shades of interpretation.
In later decades, as computing power grew, researchers switched to using statistics to extract patterns from massive quantities of data. These methods are often referred to as ‘machine learning’. Rather than trying to encode high-level knowledge and logical reasoning, machine learning employs a bottom-up approach in which algorithms discern relationships by repeating tasks, such as classifying the visual objects in images or transcribing recorded speech into text. Such a system might learn to identify images of cats, for example, by looking at millions of cat photos, or to make a connection between cats and mice based on the way they are referred to throughout large bodies of text.
Machine learning has produced many tremendous practical applications in recent years. We’ve built systems that surpass us at speech recognition, image processing and lip reading; that can beat us at chess, Jeopardy! and Go; and that are learning to create visual art, compose pop music and write their own software programs. To a degree, these self-teaching algorithms mimic what we know about the subconscious processes of organic brains. Machine-learning algorithms start with simple ‘features’ (individual letters or pixels, for instance) and combine them into more complex ‘categories’, taking into account the inherent uncertainty and ambiguity in real-world data. This is somewhat analogous to the visual cortex, which receives electrical signals from the eye and interprets them as identifiable patterns and objects.
But algorithms are a long way from being able to think like us. The biggest distinction lies in our evolved biology, and how that biology processes information. Humans are made up of trillions of eukaryotic cells, which first appeared in the fossil record around 2.5 billion years ago. A human cell is a remarkable piece of networked machinery that has about the same number of components as a modern jumbo jet – all of which arose out of a longstanding, embedded encounter with the natural world. In Basin and Range (1981), the writer John McPhee observed that, if you stand with your arms outstretched to represent the whole history of the Earth, complex organisms began evolving only at the far wrist, while ‘in a single stroke with a medium-grained nail file you could eradicate human history’.
The traditional view of evolution suggests that our cellular complexity evolved from early eukaryotes via random genetic mutation and selection. But in 2005 the biologist James Shapiro at the University of Chicago outlined a radical new narrative. He argued that eukaryotic cells work ‘intelligently’ to adapt a host organism to its environment by manipulating their own DNA in response to environmental stimuli. Recent microbiological findings lend weight to this idea. For example, mammals’ immune systems have the tendency to duplicate sequences of DNA in order to generate effective antibodies to attack disease, and we now know that at least 43 per cent of the human genome is made up of DNA that can be moved from one location to another, through a process of natural ‘genetic engineering’.
Now, it’s a bit of a leap to go from smart, self-organising cells to the brainy sort of intelligence that concerns us here. But the point is that long before we were conscious, thinking beings, our cells were reading data from the environment and working together to mould us into robust, self-sustaining agents. What we take as intelligence, then, is not simply about using symbols to represent the world as it objectively is. Rather, we only have the world as it is revealed to us, which is rooted in our evolved, embodied needs as an organism. Nature ‘has built the apparatus of rationality not just on top of the apparatus of biological regulation, but also from it and with it’, wrote the neuroscientist Antonio Damasio in Descartes’ Error (1994), his seminal book on cognition. In other words, we think with our whole body, not just with the brain.
I suspect that this basic imperative of bodily survival in an uncertain world is the basis of the flexibility and power of human intelligence. But few AI researchers have really embraced the implications of these insights. The motivating drive of most AI algorithms is to infer patterns from vast sets of training data – so it might require millions or even billions of individual cat photos to gain a high degree of accuracy in recognising cats. By contrast, thanks to our needs as an organism, human beings carry with them extraordinarily rich models of the body in its broader environment. We draw on experiences and expectations to predict likely outcomes from a relatively small number of observed samples. So when a human thinks about a cat, she can probably picture the way it moves, hear the sound of purring, feel the impending scratch from an unsheathed claw. She has a rich store of sensory information at her disposal to understand the idea of a ‘cat’, and other related concepts that might help her interact with such a creature.
This means that when a human approaches a new problem, most of the hard work has already been done. In ways that we’re only just beginning to understand, our body and brain, from the cellular level upwards, have already built a model of the world that we can apply almost instantly to a wide array of challenges. But for an AI algorithm, the process begins from scratch each time. There is an active and important line of research, known as ‘inductive transfer’, focused on using prior machine-learned knowledge to inform new solutions. However, as things stand, it’s questionable whether this approach will be able to capture anything like the richness of our own bodily models.
On the same day that SwiftKey unveiled Hawking’s new communications system in 2014, he gave an interview to the BBC in which he warned that intelligent machines could end mankind. You can imagine which story ended up dominating the headlines. I agree with Hawking that we should take the risks of rogue AI seriously. But I believe we’re still very far from needing to worry about anything approaching human intelligence – and we have little hope of achieving this goal unless we think carefully about how to give algorithms some kind of long-term, embodied relationship with their environment.